Keep Your Transformation on Track
How a Data Strategy Powers Your Artificial Intelligence Journey
The Change Agent
Data-driven decision-making is a cornerstone of every modern business strategy. By leveraging data-driven insights, organizations can optimize processes, enhance customer experiences, make informed decisions, and even predict future events.
Data in Motion
In today’s rapidly evolving digital landscape, business use cases for AI technologies have sparked a wave of fervor among Fortune 500 companies. Mentions of AI on earnings calls are up over 100% compared with those from a year ago.1 Advanced technologies like AI promise to automate complex tasks, predict consumer behavior and enable data-driven decision-making. However, the efficacy of AI hinges on an often underestimated factor: a data strategy.
A well-crafted data strategy serves as a North Star, guiding organizations toward successfully realizing the full potential of AI and achieving transformative business outcomes through data-driven transformation. In a field that’s growing more crowded by the day, successful organizations are those that consider the significance of a data strategy and its pivotal role in driving successful AI adoption.
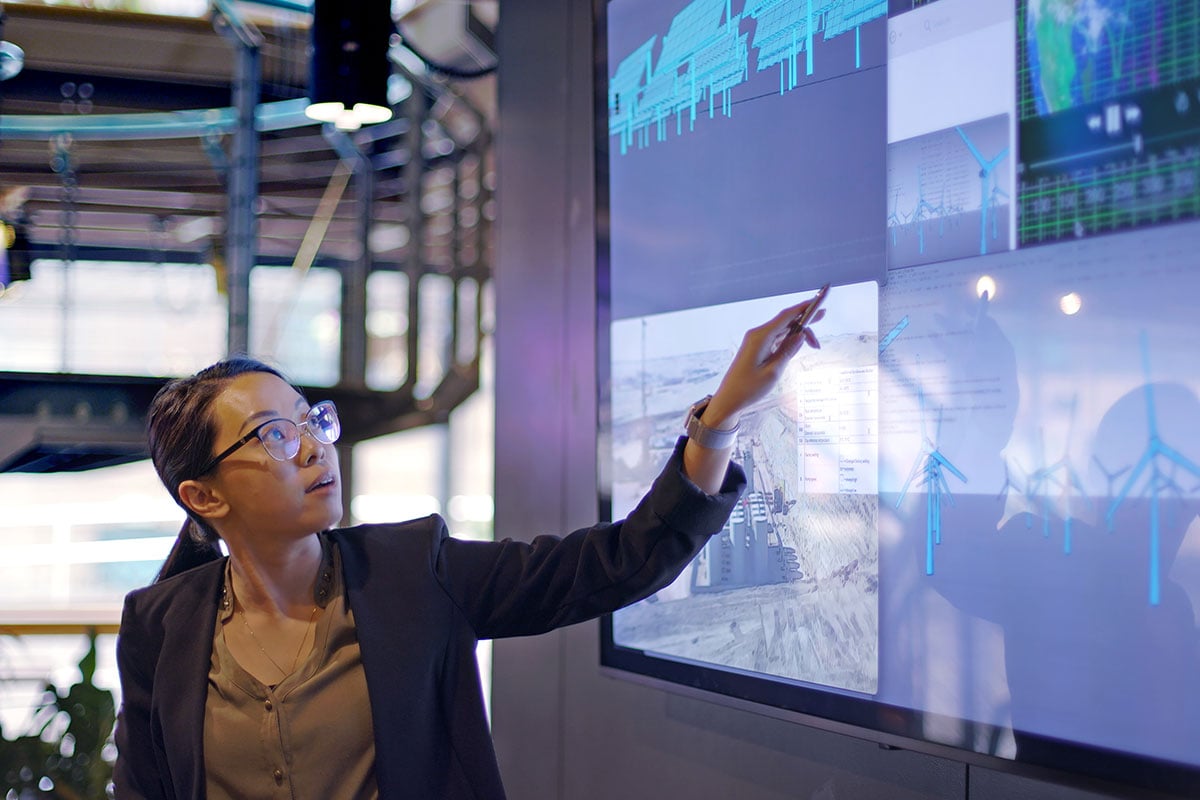
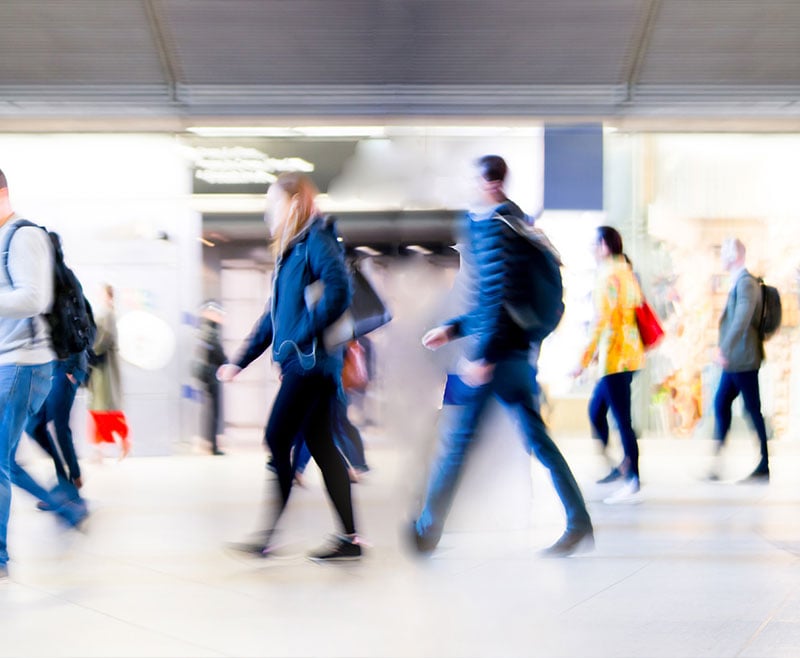
At its core, a data strategy is a comprehensive plan that outlines how an organization acquires, stores, manages and uses data to achieve its objectives. Data-driven decision-making is a cornerstone of every modern business strategy. By leveraging data-driven insights, organizations can optimize processes, enhance customer experiences, make informed decisions and even predict future events. However, a significant amount of valuable information remains dormant and untapped within organizations. This dormant data often exists in disparate systems or unstructured formats or simply lacks integration. The challenge lies in converting this dormant data to an active, usable state.
To address this, organizations are increasingly investing in advanced data analytics, machine learning and AI. These technologies help unearth insights from an organization’s internal and external data by identifying patterns, correlations and trends that might otherwise go unnoticed. By transforming data into actionable insights, businesses can make more informed decisions, optimize processes, enhance customer experiences and gain a competitive edge.
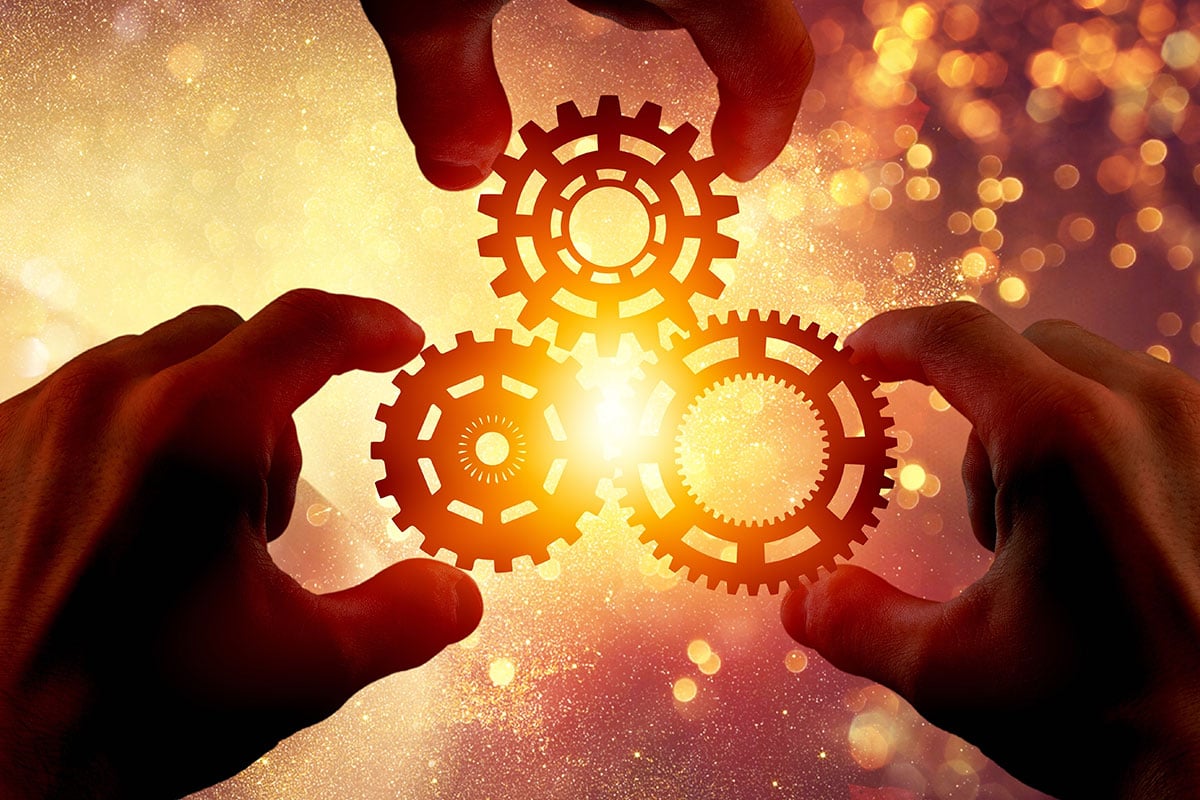
Unlocking the potential of data involves implementing robust data integration strategies, enhancing data quality and creating an organizational culture that values data-driven insights. Organizations that prioritize data activation can harness the full spectrum of their information resources, enabling them to make more proactive, agile and effective decisions in today’s rapidly evolving business landscape. There are a number of industry sectors where a holistic data strategy is driving AI insights and leading overall data-driven transformation.
- In healthcare, a data strategy enables AI applications like predictive analytics to forecast disease outbreaks, personalize treatment plans and improve patient outcomes. This approach mitigates challenges, such as resource allocation inefficiencies, and enhances overall healthcare delivery.
- In finance, data-driven AI algorithms analyze market trends, customer behaviors and risk factors to optimize investment strategies, detect fraudulent activities and predict market fluctuations. This helps financial institutions navigate volatile markets and ensure regulatory compliance while improving risk management practices.
- In e-commerce and retail, AI-powered recommendation systems driven by data strategies can provide beneficial information for a better customer experience. These systems personalize product suggestions, enhancing user experiences and driving sales. Data-driven insights also enable inventory optimization, ensuring that products are available when and where customers demand them.
In an era where artificial intelligence is poised to reshape industries, organizations cannot afford to overlook the role of a data strategy. It is the linchpin that ensures AI initiatives deliver on their promises. From data collection to model training and ongoing observation, a comprehensive AI data strategy aligns data practices with business goals, safeguarding against pitfalls and maximizing potential value. As organizations embark on their AI journeys, a solid data analytics strategy is not just an option—it’s the driving force behind success in the age of data-driven transformation.
Market Perspective
AWS Principal Data Strategist, Kathy Koontz shares her point of view on generative AI and how organizations can create business value.
Generative AI: The New Age of Artificial Intelligence
How would you define generative AI and its significance in the field of artificial intelligence?
Kathy Koontz (AWS): Generative AI didn’t come out of nowhere. Machine learning research goes back decades. Across our organization, generative AI is used for personalization on the Amazon retail site. It’s used to control robotics in our fulfillment centers. It’s used by Alexa to improve intent recognition and speech synthesis. Machine learning is in Amazon’s DNA. Generative AI is a type of artificial intelligence or machine learning that can create new content and ideas, including conversations, stories, images, videos and music. It’s powered by large models that are pretrained on vast amounts of data. These are commonly referred to as “foundation models.” And what makes foundation models special is that they can perform many tasks because they contain a large number of parameters that make them capable of learning complex concepts. But at its core, it still comes back to the basic analytics of prediction. It’s just predicting much more complex concepts that thereby give it application in a much wider variety of tasks.
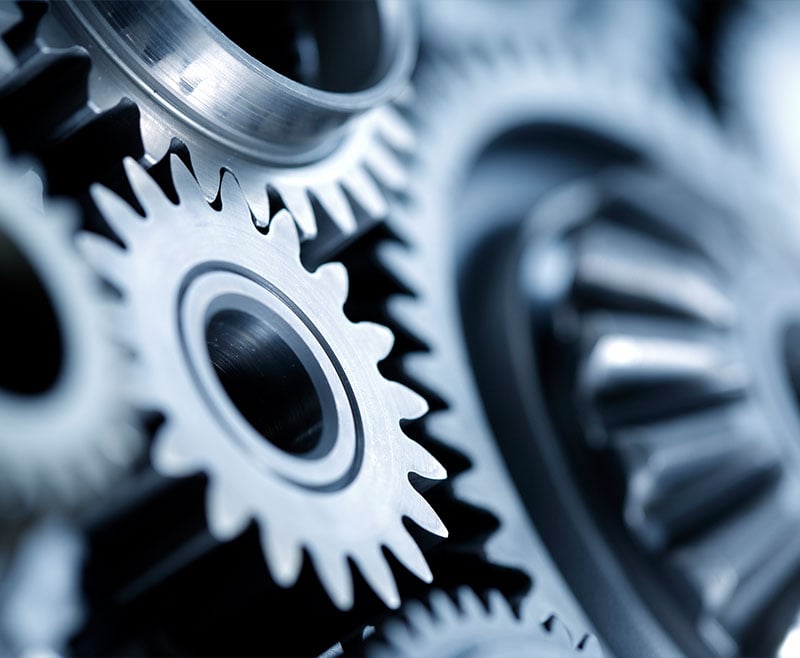
What are some of the challenges or risks associated with generative AI that businesses must keep in mind?
KK (AWS): There are a lot of risks, but they really fall into two interlinked categories: ethics and privacy. And that’s been common in the usage of data since personalized marketing started. With personalization, you can see usages of data that create that creep factor. Well, generative AI can do that much more quickly and much more broadly. So as it relates to ethics, organizations need to align their AI output with their overall ethical position, their beliefs and their brand voice. It’s important to utilize basic reinforcement learning where you apply human feedback on the AI output to reinforce the appropriate outcomes and penalize those that are not. And this needs to be an ongoing effort, not a one-and-done activity. It comes down to ensuring that the output from your Gen AI is factual and free from what is referred to as hallucinations. Frankly, that’s just table stakes. Ensuring that the output is an expression of your organization and how you want to be perceived in the marketplace is critical. The way to get there is to leverage the organization’s own data to fine-tune the model, which really accelerates that alignment with data privacy. An organization’s data is one of the few proprietary assets it has left. The data must be kept secure in its own environment rather than fed into an open-source model that could be potentially used by a competitor or a disruptive market entrant or [could be] even releasing an organization’s proprietary data into the wild of open source. To help mitigate that risk—what we see as a best practice—organizations must think about bringing the model to the data and not the other way around.
Generative AI is a type of artificial intelligence or machine learning that can create new content and ideas (...) but at its core, it still comes back to the basic analytics of prediction.
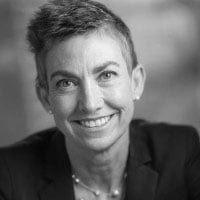
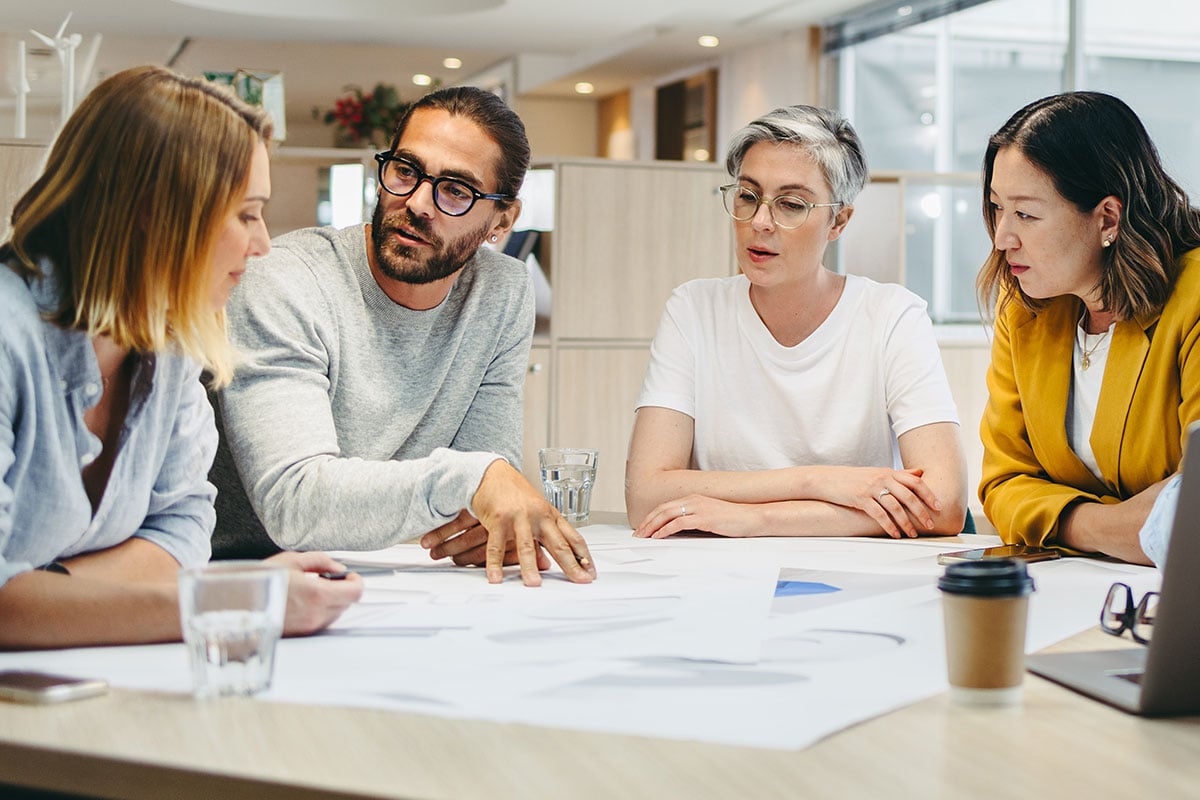
What are some real-world applications of generative AI that you find particularly promising?
KK (AWS): Generative AI really falls into four main areas. There’s creative output that involves writing code, designing and modeling, which is often being used behind the scenes to personalize search or summarize complex subject matter. It can also help in new domain exploration by answering much more complex questions than what you see with traditional chatbots. It can aid in onboarding new team members into potentially complex subject areas where the new employee can have a much more question-and-answer or conversational-type approach to learning new subjects. And then there’s expert decision-making support where the model is providing suggestions and automating complex tasks to really save the human cognitive power for those things that require human intelligence by reducing the consideration set of things that they may need to consider.
Let’s say you want to create an onboarding form for customers who are interested in joining a product beta program. You can create what we call skeleton generation. You can ask the model to create the form and then apply changes to fit your exact need. You can then get more specific results by telling the model exactly what the form needs to include, such as customer contact details, their industry or their intended use case.
Organizations are also using generative AI to search, find and synthesize information from a large corpus of data. Let’s say a company is onboarding a maintenance engineer. They can train a model on all of the technical manuals, the field notes, the service tickets and the customer center interactions so that the maintenance engineer could then ask questions of generative AI as it encounters different challenges or opportunities at a customer site.
Our Perspective
TEKsystems leaders Srinivasan Swaminatha and Ramesh Vishwanathan share their points of view on how organizations can leverage automation to enhance customer experiences, reduce operating costs and increase profitability.
Strategizing the Data Revolution
Recent decades have proven the remarkable potential of data. This encompasses activities involving small data, big data, statistics, analytics and AI, undertaken by employees across all levels of an organization. Such endeavors empower businesses to each enhance their performance in diverse ways. On the one hand, the focal point is AI, particularly generative AI, which has the potential to revolutionize the business landscape and is generating significant excitement. On the other hand, even basic analyses using modest data volumes are astonishingly effective at aiding companies in optimizing decisions, refining business processes, gaining deeper customer insights and elevating products and services in response to customer demands. A quick scan of popular media might lead one to believe that data, analytics and AI are orchestrating a global takeover. But how do organizations seize upon this opportunity and use it to their advantage?
Every organization has likely already embarked on a data journey. Their starting point depends on their past trajectory and the foundational work that has already been done. A winning data strategy must encompass the full spectrum of back-end technology, front-end strategy and all elements in between. Regardless of where the organizations are on this journey, the first crucial step is digitalization. We generate petabytes of data each hour, and yet many processes still hinge on paper-based information. Consider a recent visit to the doctor, where filling out a paper form with personal details and medical history was necessary. While this information is critical for medical staff, its storage and security remain opaque. Before an organization can unlock potent data insights, it must begin by digitizing its business processes.
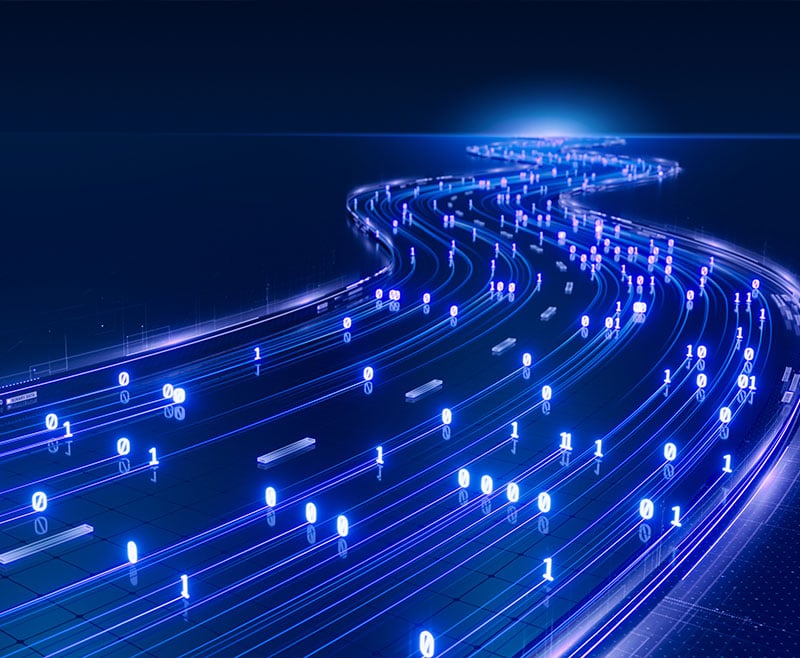
The promise of AI is very real. But companies can get caught up in the hype. First, they must consider their data strategy which can’t just be an IT initiative or siloed in different functions of the business. They need a holistic data strategy approach that takes into account their entire ecosystem, including their internal data and any external data required before they’re able to realize the transformational value of AI.
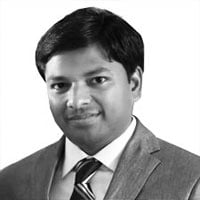
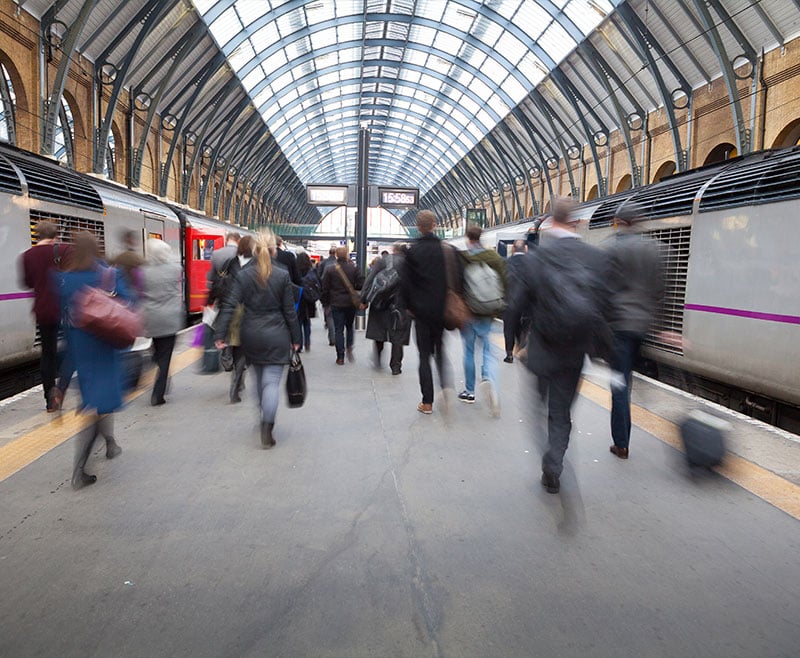
Subsequently, the journey evolves into a sequence of establishing the right foundation that empowers users to make decisions grounded in data. The organization collects, stores, archives and governs data while ensuring the framework of its modern data platform is well-established. Effective data governance is critical. Without robust data governance, the organization lacks a single source of truth from which strategic decisions can be derived. At this phase, much of the decision-making remains informed by reactive analytics—responses to events that have already happened. Users analyze year-over-year or quarter-over-quarter trends to glean insights from what’s occurred. This certainly holds value, but the organization has yet to reach a state of agility where it can anticipate and quickly react to future and emergent developments. Data monetization and transformation into an organizational asset remain unrealized.
Governance and Ethical Considerations
Artificial intelligence initiatives require access to vast amounts of data, necessitating scalable and efficient data storage solutions. A data strategy outlines the infrastructure needed to store, manage, and retrieve data seamlessly. Organizations must weigh the pros and cons of cloud-based and on-premises storage, considering factors such as cost, scalability, and data governance.
As data becomes the lifeblood of AI initiatives, governance, data security and privacy concerns come to the forefront. A comprehensive data strategy includes measures to safeguard sensitive data, adhere to regulatory standards, and address ethical considerations. Organizations need to strike a balance between data openness for AI and ensuring data protection.
The realm of artificial intelligence is one of constant evolution. As algorithms learn and adapt, your data strategy must evolve alongside them.
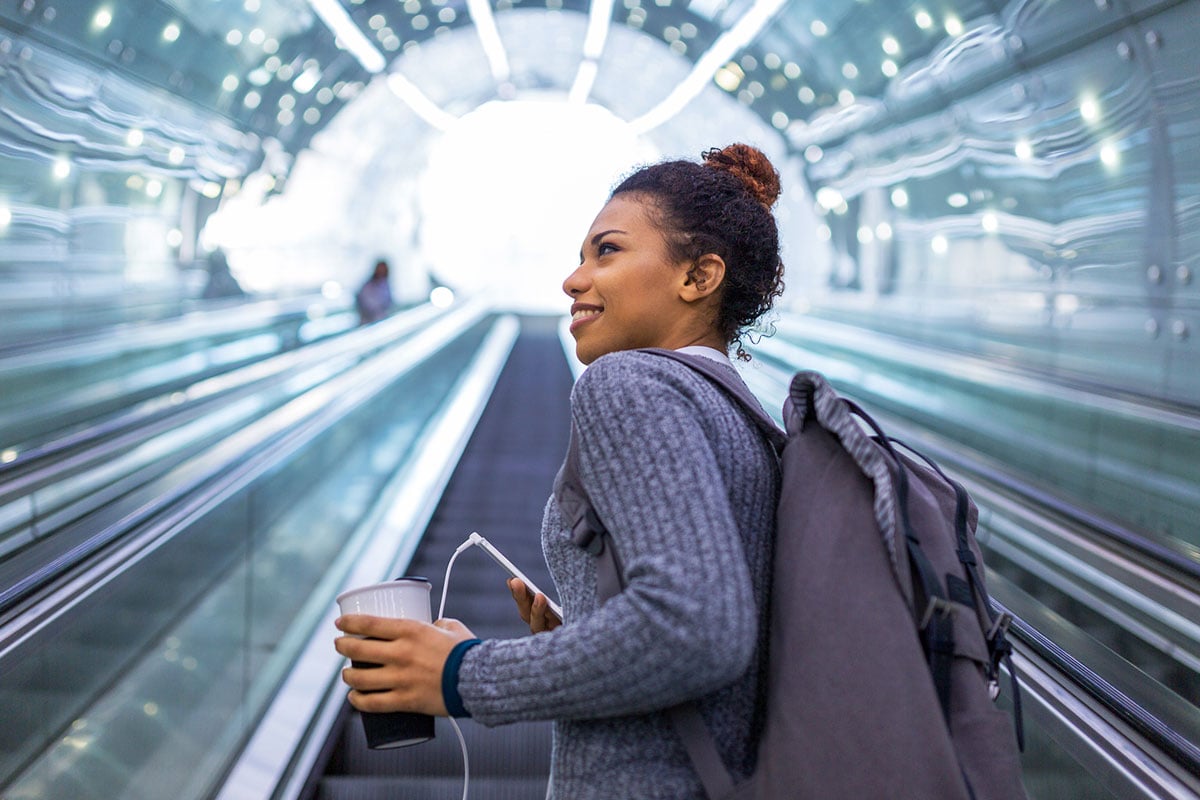
By bringing together databases, analytics, AI and machine learning, successful organizations revolutionize the enterprise and deliver value across the life cycle of their data platform. Users gain unprecedented access to make data-driven strategic decisions, enhancing customer service and optimizing operations through intelligent analytics. At this stage of the journey, data emerges as a prized asset. Across the enterprise, business users harness high-quality, reliable and timely data to uncover new revenue streams, enhance customer experiences and propel organizational growth.
Developing an effective data analytics strategy takes time and effort. Stay focused on your objectives and be prepared to iterate and adjust your approach as you learn from your experiences. By integrating AI considerations into your data strategy, you can harness the power of AI to drive innovation, improve efficiency and gain competitive advantages while maintaining ethical and responsible AI practices.
Before you begin working with different data tools, you must ask yourself how you can achieve repeatability. How can you put the process into multiple different business use cases or business needs for the long-haul? How can you make the data work best for you?
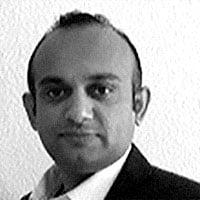
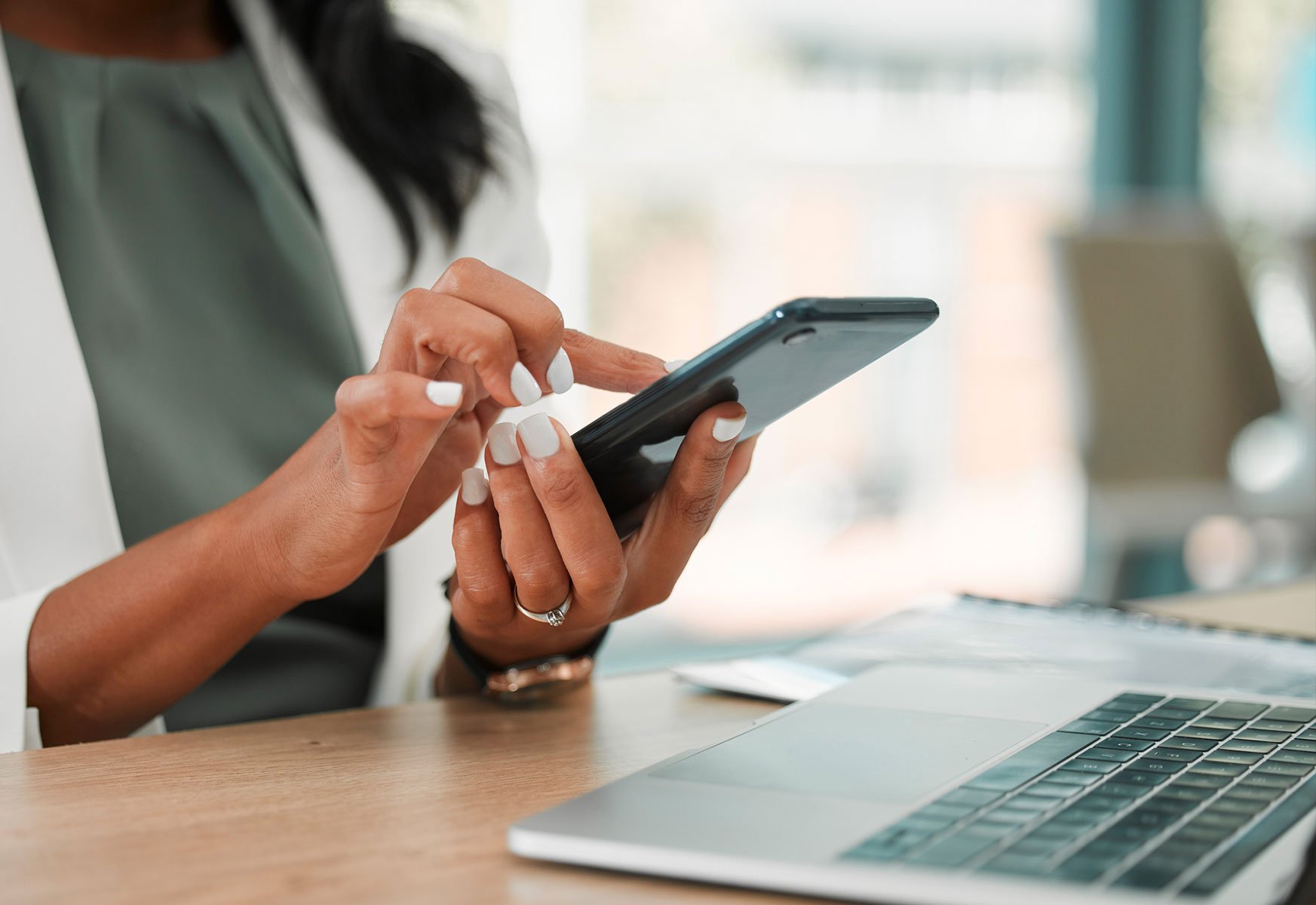
Real-World Application: Nike
When one thinks of sports apparel, the name that inevitably springs to mind is Nike. For over 50 years, Nike has stood as an industry juggernaut, renowned for their unwavering commitment to innovation. However, what makes Nike’s recent transformation particularly intriguing is their departure from their traditional business model.
Historically, Nike has thrived on a retail-first approach, with the majority of their revenue stemming from wholesale operations. While this aspect remains integral, Nike has made significant strides in the realm of direct-to-consumer sales. In 2018, Nike Direct, the company’s direct-to-consumer initiative, contributed a noteworthy $10 billion to their sales revenue. Fast forward to 2023, and Nike Direct has doubled its impact, boasting an impressive $21.3 billion in revenues.
The key to Nike’s triumph in direct-to-consumer ventures lies in their data-driven approach to enhancing the customer experience. Central to this strategy is the Nike app, which not only strengthens the brand’s connection with their customer base but also provides exclusive benefits. Through the Nike Plus rewards program, users gain access to early product launches and unique offers. The differentiation extends further with access to Nike’s team of experts tailored to individual sports preferences. Whether you’re a runner in need of the perfect shoe or a fitness enthusiast seeking personalized workouts, the Nike app has it covered. Moreover, users enjoy priority access to Nike’s exclusive events, making them feel part of an exclusive community.
Nike’s acquisition of Zodiac, a data analytics company, has been instrumental in this transformation. By mining insights from user data gathered through the app and devices like Fitbits, Nike gains profound insights into user habits and purchasing patterns. Armed with this data, Nike fine-tunes their customer acquisition and retention strategies. For instance, if a user typically buys shoes every eight months but has not made a purchase in over a year, Nike proactively engages them, prompting a new purchase.
This data-driven approach has not only improved customer acquisition and retention but also enabled Nike to reduce churn, a significant cost-savings measure. As competition from startups continues to disrupt the apparel industry, Nike’s commitment to data science stands as their defense strategy. Nike Direct is not merely an app; it embodies the company’s mission to intimately understand their customers and deliver superior products and services. In a world where data reigns supreme, Nike’s shift toward a more personalized and data-driven customer experience reflects their dedication to remaining at the forefront of the sports apparel industry.
All information shared herein was accessed from public sources as indicated and is not indicative that the named entity is a TEKsystems client nor that the work was performed by TEKsystems.